Steps: [ install | jax | haiku | q-learning | dqn | ppo | next_steps ]
DQN on CartPole¶
In this example we build a slightly more sophisticated agent known as DQN (paper).
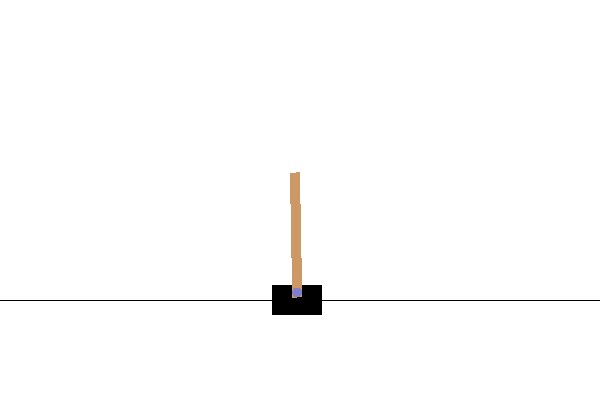
You’ll solve the CartPole environment, in which the agent is tasked to balance an pole fixed to a cart. The way the agent does this is by nudging the cart either to the left or the right at every time step.
This example is nice, because it shows a few more components. Most notably, it introduces the notion of a target network and the use of an experience-replay buffer.
Just as in the first example, you may either hit the Google Colab button or download and run the script on your local machine.
import coax
import gymnasium
import haiku as hk
import jax
import jax.numpy as jnp
from coax.value_losses import mse
from optax import adam
# the name of this script
name = 'dqn'
# the cart-pole MDP
env = gymnasium.make('CartPole-v0', render_mode='rgb_array')
env = coax.wrappers.TrainMonitor(env, name=name, tensorboard_dir=f"./data/tensorboard/{name}")
def func(S, is_training):
""" type-2 q-function: s -> q(s,.) """
seq = hk.Sequential((
hk.Linear(8), jax.nn.relu,
hk.Linear(8), jax.nn.relu,
hk.Linear(8), jax.nn.relu,
hk.Linear(env.action_space.n, w_init=jnp.zeros)
))
return seq(S)
# value function and its derived policy
q = coax.Q(func, env)
pi = coax.BoltzmannPolicy(q, temperature=0.1)
# target network
q_targ = q.copy()
# experience tracer
tracer = coax.reward_tracing.NStep(n=1, gamma=0.9)
buffer = coax.experience_replay.SimpleReplayBuffer(capacity=100000)
# updater
qlearning = coax.td_learning.QLearning(q, q_targ=q_targ, loss_function=mse, optimizer=adam(0.001))
# train
for ep in range(1000):
s, info = env.reset()
# pi.epsilon = max(0.01, pi.epsilon * 0.95)
# env.record_metrics({'EpsilonGreedy/epsilon': pi.epsilon})
for t in range(env.spec.max_episode_steps):
a = pi(s)
s_next, r, done, truncated, info = env.step(a)
# extend last reward as asymptotic best-case return
if truncated:
r = 1 / (1 - tracer.gamma) # gamma + gamma^2 + gamma^3 + ... = 1 / (1 - gamma)
# trace rewards and add transition to replay buffer
tracer.add(s, a, r, done or truncated)
while tracer:
buffer.add(tracer.pop())
# learn
if len(buffer) >= 100:
transition_batch = buffer.sample(batch_size=32)
metrics = qlearning.update(transition_batch)
env.record_metrics(metrics)
# sync target network
q_targ.soft_update(q, tau=0.01)
if done or truncated:
break
s = s_next
# early stopping
if env.avg_G > env.spec.reward_threshold:
break
# run env one more time to render
coax.utils.generate_gif(env, policy=pi, filepath=f"./data/{name}.gif", duration=25)